CH 5 Predicting single unit responses in macaque V1
The contents of this chapter are available in Using deep learning to probe the neural code for images in primary visual cortex3
Abstract
Primary visual cortex (V1) is the first stage of cortical image processing, and major effort in systems neuroscience is devoted to understanding how it encodes information about visual stimuli. Within V1, many neurons respond selectively to edges of a given preferred orientation: These are known as either simple or complex cells. Other neurons respond to localized center–surround image features. Still others respond selectively to certain image stimuli, but the specific features that excite them are unknown. Moreover, even for the simple and complex cells—the best-understood V1 neurons—it is challenging to predict how they will respond to natural image stimuli. Thus, there are important gaps in our understanding of how V1 encodes images. To fill this gap, we trained deep convolutional neural networks to predict the firing rates of V1 neurons in response to natural image stimuli, and we find that the predicted firing rates are highly correlated (CCnorm = 0.556 ± 0.01) with the neurons’ actual firing rates over a population of 355 neurons. This performance value is quoted for all neurons, with no selection filter. Performance is better for more active neurons: When evaluated only on neurons with mean firing rates above 5 Hz, our predictors achieve correlations of CCnorm = 0.69 ± 0.01 with the neurons’ true firing rates. We find that the firing rates of both orientation-selective and non-orientation-selective neurons can be predicted with high accuracy. Additionally, we use a variety of models to benchmark performance and find that our convolutional neural-network model makes more accurate predictions.
5.1 Introduction
Our ability to see arises because of the activity evoked in our brains as we view the world around us. Ever since Hubel and Wiesel (1959) mapped the flow of visual information from the retina to thalamus and then cortex, understanding how these different regions encode and process visual information has been a major focus of visual systems neuroscience. In the first cortical layer of visual processing—primary visual cortex (V1)—Hubel and Wiesel identified neurons that respond to oriented edges within image stimuli. These are called simple or complex cells, depending on how sensitive their responses are to shifts in the position of the edge. The simple and complex cells are well studied (Lehky, Sejnowski, & Desimone, 1992; David, Vinje, & Gallant, 2004; Montijn, Meijer, Lansink, & Pennartz, 2016). However, many V1 neurons are neither simple nor complex cells, and the classical models of simple and complex cells often fail to predict how those neurons will respond to naturalistic stimuli (Olshausen & Field, 2005). Thus, much of how V1 encodes visual information remains unknown. We use deep learning to address this longstanding problem.
5.2 Methods
5.2.1 Experimental Data
Neural activity was recorded in monkeys’ V1 as they were shown a series of images (Fig 5.1A). The image set contains 270 circularly cropped natural images (Fig 5.1B). (C) The response of a single neuron over repeated presentations of an image. Ticks indicate the neuron’s spiking; each row corresponds to a different image-presentation trial. During the response window, the firing rate is computed and then averaged over trials to yield the average response An,i used in our analysis. (D) The neuron responds to image stimuli with a latency of ∼50 ms from the image onset at t = 0, as seen in the peristimulus time histogram (firing rate plotted against time, averaged over all 270 images).
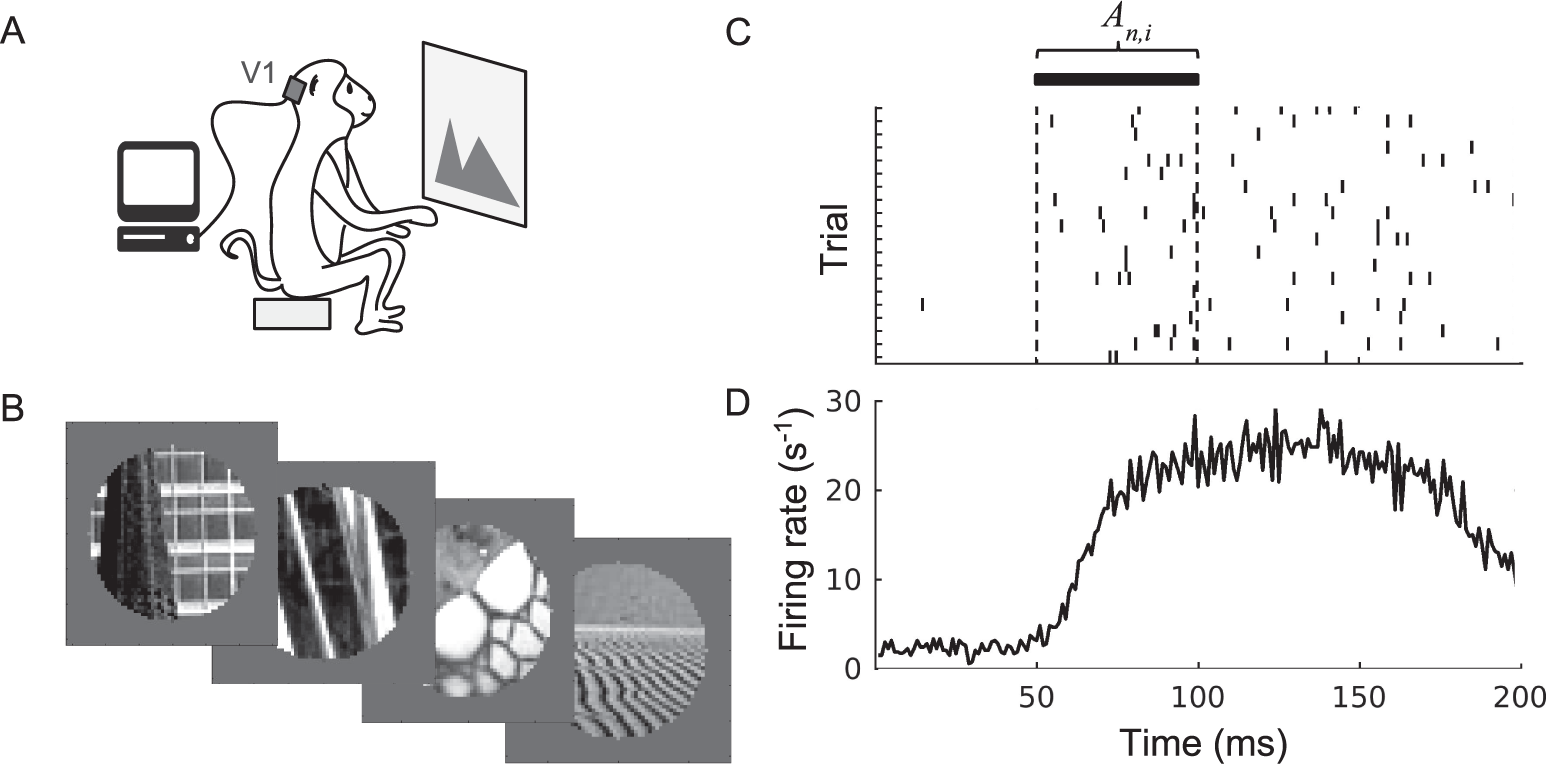
Figure 5.1: Experimental data collection and processing.